In inferential statistics, a result is statistically significant when it is judged as unlikely to have occurred by sampling error alone.
Statistical software packages generally report statistical significance using a test statistic and a p (probability) value (ranging between 0 and 1). If the p-value is less than a pre-selected critical value (critical α) then in classical test theory it is considered to be "statistically significant".
The greater the statistical power, the greater the likelihood of a test being statistically significant.
Logic
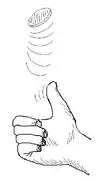
If you were betting on coin tosses, how many heads in a row would someone else need to throw before you'd protest that something “wasn't right” (i.e., there was bias - it isn't a 50-50 coin)?
This is the logic as significance testing - basically, how unlikely would a set of results need to be before you'd conclude that it is different to one's expectations?
See also: A Coin-Flipping Exercise to Introduce the P-Value
Based on the distributional properties of a sample dataset, we can extrapolate (guessestimate) about the probability of the observed differences or relationships existing in a population. In doing this, we are assuming that the sample data is representative and that data meets the assumptions associated with the inferential test.
A null hypothesis (H0) states the expected effect in the population (or no effect). A p-value is obtained from sample data to determine the likelihood of H0 being true. Researcher tolerates some false positives (Type I error; critical α) in order to make a decision about H0
History
(NRCS_Photo_Gallery).jpg.webp)
Significant testing evolved during the 20th century and become an important scientific methodology.
Karl Pearson laid the foundation for ST as early as 1901 (Glaser, 1999).
Sir Ronald Fisher 1920’s-1930’s (1925) developed significant testing for agricultural data to help determine agricultural effectiveness e.g., whether plants grew better using fertilizer A vs. B. The method was used to test whether variation in agricultural output were due to chance or not.
Agricultural research designs couldn’t be fully experimental because variables such as weather and soil quality couldn't be fully controlled, therefore it was needed to determine whether variations in the DV were due to chance or the IV(s).
Significance testing spread to other fields, including social sciences. The spread was aided by the development of computers and statistical method training.
Criticisms
The use of significance testing was critiqued as early as 1930. Cohen, in particular, provided a substantial critique during the 1980’s and 1990’s of the widespread use of Null Hypothesis Significance Testing (NHST), including over-use and mis-use. This lead to a critical mass of awareness and changes were made to publication guidelines and teaching during the 2000s to avoid over-reliance on significant testing and to encourage use of alternative and adjunct techniques, including consideration of effect sizes, confidence intervals and statistical power.
The key criticisms include:
- The null hypothesis is rarely true
- Significance testing only provides a binary decision (yes or no) and the direction of the effect - but mostly we are interested in the size of the effect – i.e., how much of an effect?
- Statistical vs. practical significance
- Sig. is a function of ES, N and critical α - e.g., can get statistical significance, even with very small population differences, if N, ES and/or critical α are large enough
An example of the criticisms include:
- "For example, Frank Yates (1951), a contemporary of Fisher, observed that the use of the null hypothesis significance test: "has caused scientific researcher workers to pay unde attention to the results of the tests of significance that they perform on their data and too little attention to the estimates of the magnitude of the effects they are investigating... The emphasis on tests of significance, and the consideration of the results of each experiment in isolation, have had the unfortunate consequence that scientific workers often have regarded the execution of a test of significance on an experiment as the ultimate objective (pp. 32-33)""Kirk, 2001, p. 213)
- A more strongly worded criticism by Paul Meehl (1978) was: "I believe that the almost universal reliance on merely refuting the null hypothesis is a terrible mistake, is basically unsound, poor scientific strategy, and one of the worst things that ever happened in the history of psychology" (p. 817)
- "The current method of hypothesis testing in the social sciences is under intense criticism, yet most political scientists are unaware of the important issues being raised. Criticisms focus on the construction and interpretation of a procedure that has dominated the reporting of empirical results for over fifty years. There is evidence that null hypothesis significance testing as practised in political science if deeply flawed and widely misunderstood. This is important since most empirical work argues the value of findings through the use of the null hypothesis significance test." (Gill, 1999, p. 647)
- “Historically, researchers in psychology have relied heavily on null hypothesis significance testing (NHST) as a starting point for many (but not all) of its analytic approaches. APA stresses that NHST is but a starting point and that additional reporting such as effect sizes, confidence intervals, and extensive description are needed to convey the most complete meaning of the results... complete reporting of all tested hypotheses and estimates of appropriate ESs and CIs are the minimum expectations for all APA journals.” (APA Publication Manual (6th ed., 2009, p. 33)
Practical significance
Statistical significance means that the observed mean differences are judged to be unlikely due to sampling error.
Practical significance is about whether the difference is large enough to be of value in a practical sense. In other words, is it an effect worth being concerned about – are these noticeable or worthwhile effects? e.g., a 5% increase in well-being probably has practical value
Recommendations
- Use traditional Fisherian logic methodology (inferential testing)
- Use alternative and complementary techniques (ESs and CIs)
- Emphasise practical significance
- Recognise merits and shortcomings of each approach
Summary
- Criticisms of NHST
- Binary decision
- Doesn't directly indicate ES
- Dependent on N, ES, and critical α
- Need to know practical significance
- Recommendations
- Use complementary or alternative techniques, including power, effect size (ES) and CIs
- Wherever a p-level is reported, also report ES, N and critical α
References
- Gill, J. (1999). The insignificance of null hypothesis significance testing. Political Research Quarterly, 52(3), 647-674.
- Kirk, R. E. (1996). Practical significance: A concept whose time has come. Educational and Psychological Measurement, 56(5), 746-759.
- Kirk, R. E. (2001). Promoting good statistical practices: Some Suggestions. Educational and Psychological Measurement, 61, 213-218. doi: 10.1177/00131640121971185
- Meehl, P. E. (1978). Theoretical risks and tabular asterisks: Sir Karl, Sir Ronald, and the slow progress of soft psychology. Journal of Consulting and Clinical Psychology, 46, 806-834.